Machine Maintenance is a critical activity for a manufacturing system. We can avoid failure by doing maintenance on time. But the major challenge is to determine the best time for maintenance.
Predictive maintenance is a type of condition-based maintenance introduced to solve this problem. It helps in finding the best time for maintenance according to machine conditions.
We suggest you also read this article on machine monitoring in manufacturing.
Predictive Maintenance Definition
Predictive maintenance is a type of condition-based maintenance where we predict the machine’s time to failure and pinpoint the problem. Therefore, engineers can schedule maintenance right before machine failure.
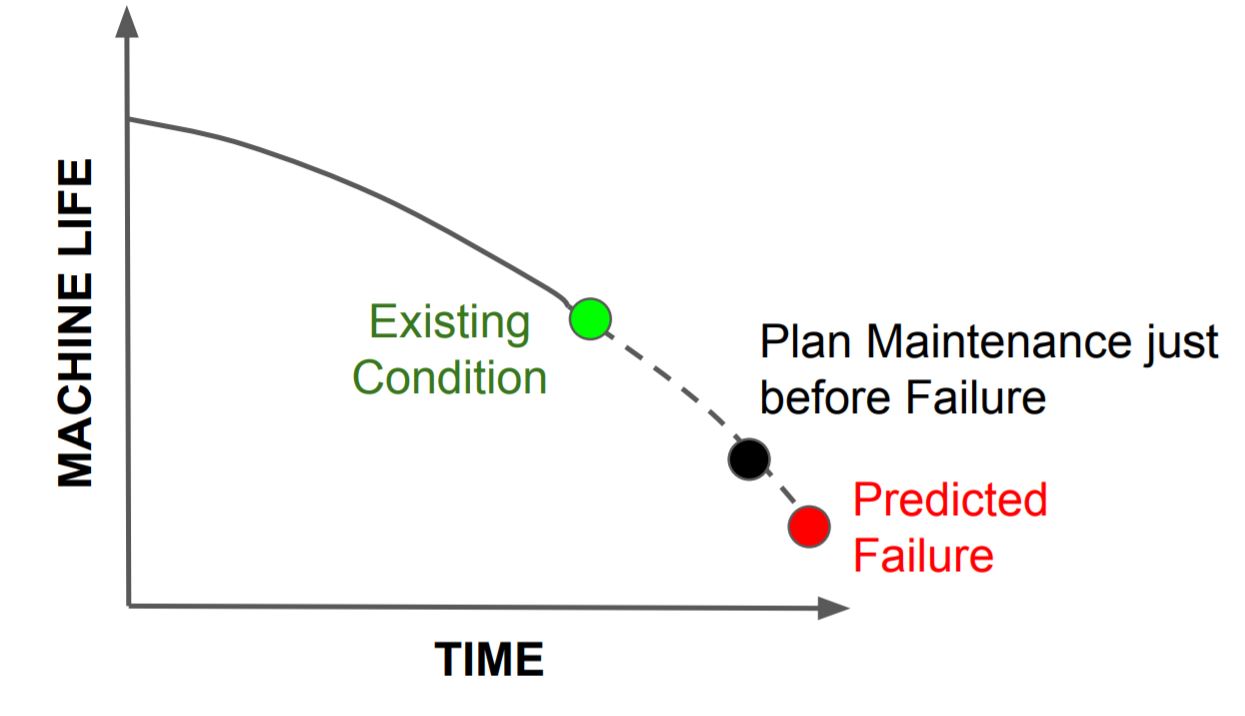
As a result, we can minimize machine downtime, and increase machine life and overall productivity.
How does Predictive Maintenance work?
In this technique, data analysis tools monitor and analyze real-time machine data to detect anomalies and possible defects in machines. We can do machine data analysis on edge computing devices or cloud computing.
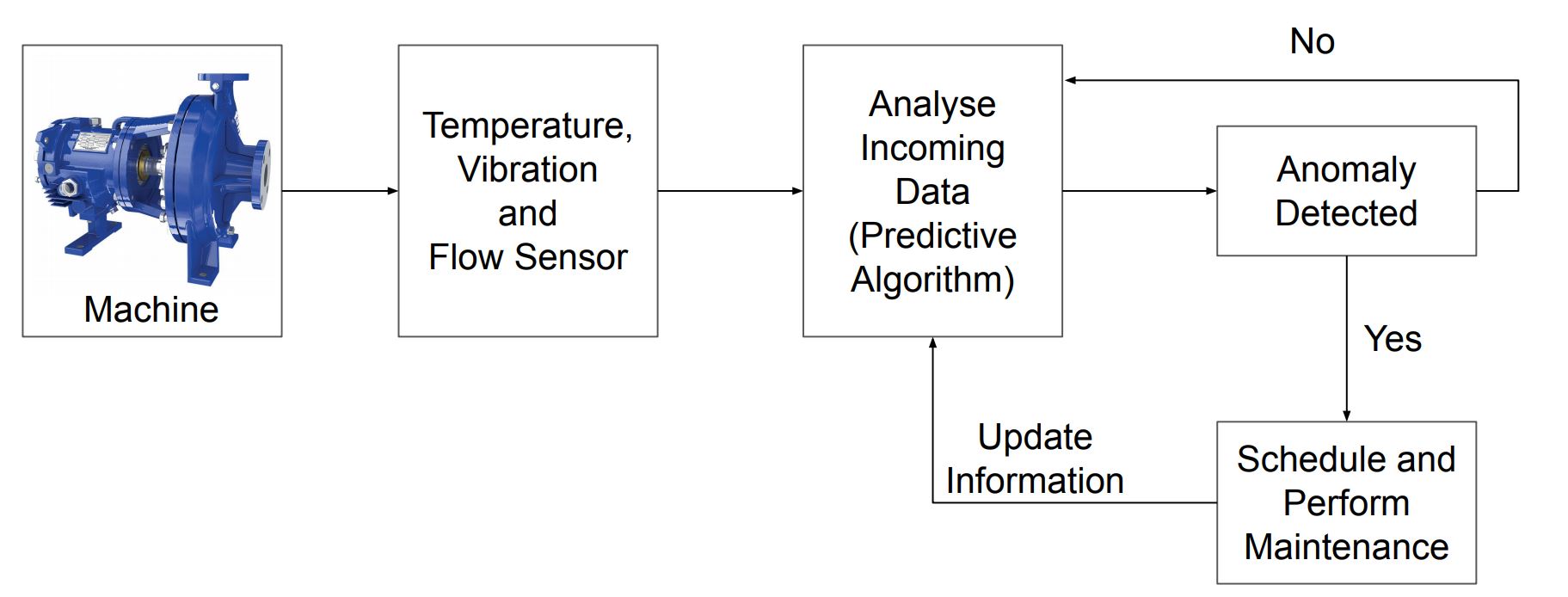
As shown in the above image, Predictive maintenance works in the following steps.
- Acquire machine data from the machine monitoring system.
- Send machine sensor data to a cloud or edge computing device.
- Analyze machine data using the predictive algorithm for anomaly detection.
- Schedule and perform the maintenance.
- Update machine maintenance information.
How to implement Predictive Maintenance
You should consider Return on investment or ROI to decide where we should implement this strategy. Mostly, it is beneficial only for critical machines and processes.
Step-1: Identify Critical Assests
Critical assets in a manufacturing unit are the assets with:
- High repairing costs.
- Frequent failures.
- Impact on production and product delivery.
For example, a turbine is a critical asset because its failure can impact electricity generation and halt production.
Step-2: Determine the Failure modes
After we identify critical assets, the next step is to determine the failure modes of that asset. Different assets can have different failure modes.
For example, a pump may fail due to a failure in bearing. On the other hand, a data center can fail due to high temperatures.
Step-3: Select the Sensors
We select the sensor according to the failure modes. Following is the list of sensors used for condition monitoring.
- Vibration Sensor
- Temperature Sensor
- Flow sensor
- Acoustical analysis
Step-4: Connect Sensors with IoT devices Acquire data in Edge computing or in Cloud
Machine sensors are connected to IoT devices to send real-time machine data to edge computing devices or the cloud.
In the cloud, machine data is processed to get insights into machine conditions. Bluetooth, Zig-bee, Wi-fi, and GPRS technologies are used to send this data to the cloud.
Step-5: Develop Predictive Algorithms
We can use machine data to develop, improve, and train the machine learning algorithm. These algorithms can predict machine life and detect the anomaly in the machine.
Step-6: Deploy the system
After the algorithm is ready and you are getting the machine data. You can deploy the program on edge computing devices or in the cloud.
Advantages and Disadvantages of Predictive Maintenance
Advantages
- Planning Machine maintenance only when the machine requires it.
- Reduced maintenance cost.
- Avoid costly breakdowns.
- Plan maintenance activities with minimal impact on production.
- Reduction in machine downtime.
Disadvantages
- High initial investment.
- Require a highly skilled workforce.
- Recommended to be used only for critical machines.
Predictive Maintenance devices and Software
Frequently Asked Questions : FAQ
All machines require maintenance to eliminate the breakdown. The cost of regular maintenance is always less than the cost of machine breakdown. Regular maintenance also ensures machines work for the maximum possible time.
Determining the best time for machine maintenance is very important. It ensures the effective utilization of resources. These techniques determine the best time for machine maintenance using artificial intelligence and machine learning techniques.
Here is the list of the most common types of maintenance techniques.
- Reactive Maintenance
- Preventive Maintenance
- Predictive Maintenance
Out of the above three maintenance techniques, condition-based maintenance is the best choice for critical machines.
In preventive maintenance, engineers decide maintenance schedules according to historical data and machine expected life. Whereas in predictive maintenance is planned according to actual machine conditions.
Condition-based maintenance is a type of predictive maintenance where machine condition determines the maintenance schedule. Whereas predictive maintenance predicts the machine condition in the future based on the machine current condition and historical available data.
Following are the application examples of predictive maintenance:
- Predict the maintenance schedule of a pump according to pump intel/outlet pressure, temperature, and electricity consumption.
- Predict a machine maintenance schedule by analyzing sensor data.
- Plan aircraft maintenance according to engine condition.
- Plan grid maintenance activities to avoid a blackout.
Add a Comment